Want to Do Corporate Innovation Right?
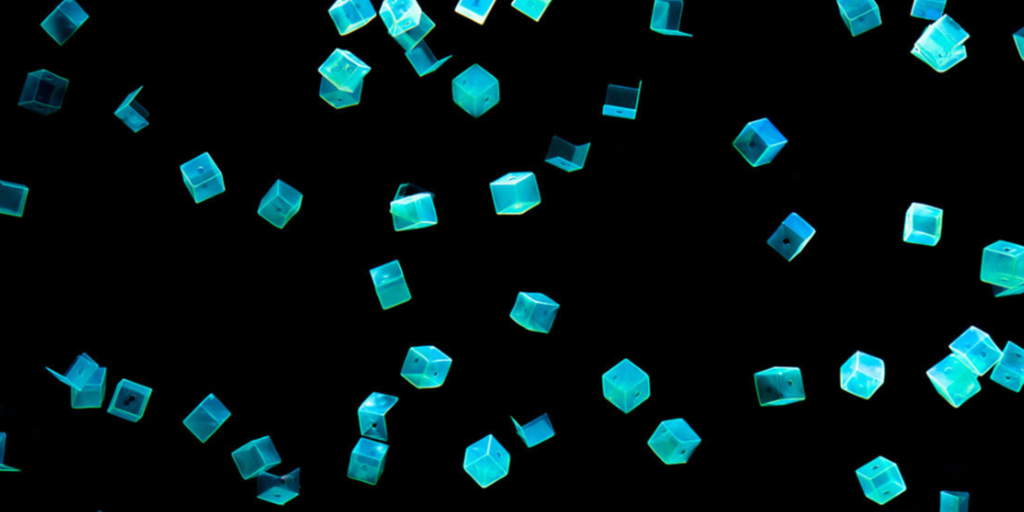
Apple fuses technology with design. IBM invests in research that is often a decade ahead of its time. Facebook “moves fast and maintains a stable infrastructure” (but apparently doesn’t break things anymore).
Each of these companies, in its own way, is a superior innovator. But what makes Google (now officially known as Alphabet) different is that it doesn’t rely on any one innovation strategy, but deploys a number of them to create an intricate — but powerful — innovation ecosystem that seems to roll out innovations by the dozens.
The company is, of course, a massive enterprise, with $75 billion in revenues, over 60,000 employees, and a dizzying array of products, from the core search business to the android operating system to nascent businesses like autonomous cars. So to better understand how Google innovates, I took a close look at what it’s doing in one area: Deep Learning, a devilishly complex form of artificial intelligence that helps machines to absorb and act on information much as humans do.
A fierce commitment to research
Like anything Google does, its Deep Learning initiative is rooted in its fierce commitment to science. The spirit of discovery is deeply embedded in Google’s DNA. After all, the company got its start from Sergey Brin and Larry Page’s NSF funded research project to catalogue the web. That passion has continued and Google invests billions of dollars every year on research and development.
Yet even that doesn’t tell the whole story. Google also routinely acquires early stage startups, many launched as a result of academic research, and invests in dozens of others through its venture capital arm. (One of Google’s freshly acquired startups, Deep Mind, recently made headlines by beating legendary Go world champion, Lee Sedol.)
Another way the company identifies and accesses breakthrough research is through its active partnership with the scientific community. It funds over 250 academic research projects a year, publishes results on public databases such as arXiv, as well as on its own research site and invites about 30 top scholars to spend sabbaticals at Google every year.
Often, top researchers come to stay. Google offers scientists such a unique research environment, with unparalleled data sets, some of the best computing architecture in the world and the opportunity to continue to publish openly, that the company is a big draw for top-notch scholars. One of these, Andrew Ng, a star in artificial intelligence circles, arrived at Google in 2010.
Innovating from the bottom up
Once he arrived at Google, Ng quickly bonded with two other researchers, Greg Corrado and Jeff Dean, who were also interested in a new brand of artificial intelligence called deep learning. Soon, the three were working together as part of their “20% time,” the firm’s well-known practice of encouraging employees to work on projects that interest them.
Some of Google’s most popular products, such as Gmail, Google News, and AdSense, originated as 20% time projects. Yet what the three had in mind was different. They weren’t interested in building products, but rather to stretch the bounds of the possible.
To understand what they were after, it helps to know something about how the brain works. When you see a friend across a crowded bar, it seems like a single event, but it isn’t. Different regions of your brain process different aspects of the experience, such as colours and shapes and integrates them into larger concepts, such as a human face, a type of hairstyle or the signature style of a popular designer.
Scientists call these different aspects “levels of abstraction.” Ordinarily, computers tend to work in a linear fashion, so have a hard time working with more than a few levels of abstraction. However, the trio thought they could do much better, expanding the hierarchy to 20 or 30 levels, which would allow for much deeper machine learning than had been previously achieved.
An internal incubator
Before long, they were making progress. As Corrado told me, “For a network to learn, you need data and Google has lots of it. The other element is really fast computing and Google’s expertise in distributed computing was also enormously helpful. So we were able to advance machine learning enormously quickly.”
About 18 months after they started, the team moved to work on their project at Google X, the company’s internal skunkworks. There, they were not only able to work on deep learning full time, but could also recruit a staff of five or six other top-notch scientists and engineers, with PhD’s in math, neuroscience, and computer science.
“The time we spent incubating in Google X was really essential,” Corrado says. “We could advance the core technology without being tied to a particular product. We went from three people working part time to eight or nine working full time. That’s the perfect size to move really fast. We were like a small startup, but had the resources of this enormous enterprise.”
The result of that incubation period was an actual product. Called DistBelief, it became their first-generation machine learning system. Before long, DistBelief was incorporated into a variety of Google products, such as Google Maps, Google Translate, and even YouTube (to help users find the videos they’re looking for).
Innovating the core
By 2012, the team had “graduated” from Google X and was named Google Brain, which has grown to around a hundred researchers at any given time, including Geoffrey Hinton, a giant in the field of deep learning, who joined in 2013. It has also built a more advanced version of DistBelief, called TensorFlow, that’s been open-sourced under an Apache license.
Today, Google Brain has become an integral part of the innovation ecosystem that spawned it. The team works actively with its internal “customers” within Google product teams to put deep learning at the centre of everything Google does. Just about anyone within Google can access Tensor Flow to make a product smarter and more effective.
For example, TensorFlow has made Google voice search faster and more accurate, reducing the error rate from around 23% to around 8%, while cutting the time to complete a search by nearly one third of a second. Errors in image search have been reduced by two thirds and users can now search unlabeled photos for everything from sunsets to specific dog breeds.
It has also led to new features, such as Smart Reply on Gmail, which offers users recommendations to respond to incoming messages. Google Translate has been expanded to 100 languages, covering 99% of the world’s population. Google Brain will most likely lead to completely new products, but it’s already showing enormous value in improving the firm’s core capabilities.
A tight feedback loop
In most organizations, innovation is treated as a fairly linear process of research, development, demonstration, and deployment, with each step acting as its silo. But at Google, everything works as a tightly coupled feedback loop, with researchers and product teams working hand in hand to not only create new products, but also to identify fruitful research areas for further study.
“Getting close to data and the real needs of users gives you the opportunity to innovate further,“ Corrado told me. He and his team work actively with not only product groups, but also fellow Googlers working on 20% projects. Rather than a group of mad scientists working on Frankenstein monsters deep in the bowels of the organization, they are active collaborators.
That’s how Google Brain filters throughout Google’s innovation ecosystem. TensorFlow provides access to basic machine learning tools, which open up new possibilities for Google’s engineers, who then reach out to the scientists within Google Brain to create new products and features. That creates a rich problem set, which helps draw top-notch researchers to Google who, in turn, create even more exciting new technology.
What makes Google special is the way it’s been able to integrate an entire portfolio of innovation strategies into a seamless whole. Product managers focus on customers needs. Researchers go where the science takes them. Engineers working on 20% time projects follow their passions. Anybody who wants to can adopt one or more of these approaches.
That takes more than a management philosophy or a streamlined operation — it requires a true spirit of discovery deeply embedded into the organization’s DNA.